How Brazil transformed Customs control through artificial intelligence and other technologies
29 October 2024
By Jorge Jambeiro Filho, Head of Innovation and Artificial Intelligence and Gustavo Lacerda Coutinho, Head of the Special Coordination for Customs Risk Management and Kelly Morgero, Head of the Mutual Recognition Agreements Department of the Authorized Economic Operator Center, Brazilian CustomsThe landscape formed by IT Customs systems in Brazil has undergone significant transformation in recent years, redefining how the Customs Administration manages and mitigates risks. Through the innovative use of artificial intelligence, including machine learning and real-time monitoring technology, Brazil has enhanced its ability to detect suspicious transactions and respond to threats, thereby strengthening the integrity of international trade operations. This article provides an update on the progress, challenges, and prospects concerning these pioneering risk management tools.
Six years have passed since Brazil’s Integrated Risk Management Solutions were first described in WCO News. Back then, the spotlight was on three key systems. The first one was SISAM[1], a machine learning system that analyzed import declarations using historical data and estimated the probability of about 30 types of errors. The second was ANIITA[2], an integrator and expert system that provided Customs officers with a unified view by combining data from various databases and applying human-defined rules to generate alerts, thus streamlining decision-making processes. The third one was PATROA, an online monitoring system that focused on real-time responsiveness, sending emails and instant messages to Customs officers whenever a suspicious transaction was detected, ensuring that immediate action could be taken when necessary.
Together, these solutions swiftly propelled the modernization of Brazil’s Customs control procedures, marking a pivotal step forward in enhancing efficiency and effectiveness. These tools have all evolved to become essential in shaping the way Brazil handles Customs operations.
ANIITA and PATROA have undergone significant upgrades, but it is SISAM whose functionalities have evolved the most. Moreover, new systems have been developed to further strengthen the country’s ability to manage and monitor international trade and passenger movements.
SISAM: assessing import declarations accurately
The Customs Selection System through Machine Learning, known as SISAM, has been instrumental in analysing all import declarations in Brazil for the last ten years. It has proven to be a powerful tool in detecting several types of errors, with the most important being the misclassification of goods. To ensure it makes use of recent technological developments, some changes have recently been made to the tool.
Leveraging infraction notices and other post-clearance decisions
The system relies on comprehensive data inputs to effectively monitor import operations. SISAM collects and analyses import declaration information which is submitted through Brazil’s Integrated International Trade System (Siscomex). The AI tool “learns” from the history of import declarations through both supervised and unsupervised learning, which are two methods through which algorithms can analyze a dataset and extract useful insights.
When an import declaration is rectified following the identification of discrepancies during clearance, the rectified version is also sent to SISAM and is used to identify direct correlations between the presence or absence of errors, and the patterns composed by the attributes of the declaration (supervised learning).
Import declarations that have been cleared without being inspected are used for unsupervised learning, and lead to the identification of typical patterns and atypical ones. The main example of this outlier detection process involves incompatibilities among the natural language description of goods and their declared nomenclature codes. Goods that are “unexpected” for a company with a certain economic activity code, and goods that are bought from suppliers or manufacturers that do not usually sell the same goods to other Brazilian importers, also draw attention.
One issue with this modus operandi was that SISAM was not making use of infraction notices which are issued when infractions are detected after clearance (as in this case the import declaration is not rectified), or of expert reports and advance rulings. To address this issue, SISAM has recently been integrated with the various systems where such information is stored, leading to the implementation of what is known as “virtual rectifications”. The official declarations are never actually rectified, but the information is part of the SISAM knowledge base.
Classification of goods, natural language processing and structured attributes
SISAM operates on a fully Bayesian model, which updates probabilities based on new evidence without full retraining. In the context of Customs operations, this allows the system to continuously refine its predictions and decision-making processes as it receives more data, allowing a prompt reaction when new frauds are encountered. Another strength of SISAM is its ability to handle wrong goods descriptions. It does this in two ways.
Firstly, it correlates the correct classification code with the originally provided description. This approach allows SISAM to become familiar with the mistakes and inaccuracies that are typically present in declarations. For instance, while the word “plastic” should, in theory, be rare in the description of a product corresponding to a NCM classification code related to rubber, SISAM recognizes that, in practice, such discrepancies are more common than one might expect.
Secondly, SISAM considers a range of additional factors beyond the goods description. These include what the importer is expected to buy, what the supplier is expected to sell, which errors in classification codes have been detected in the past, which errors in descriptions have been identified in the past, who has committed those errors, and the specific timeframes in which they occurred. By analysing these tendencies and patterns, SISAM can better predict potential discrepancies, identify anomalies more accurately, and flag suspicious transactions.
To identify valid tendencies for an importer, SISAM leverages the history of all inspected and non-inspected declarations and the history of declarations of other importers, while deliberately ignoring the non-inspected declarations of the same importer. This ability to exclude the target importer’s unverified data is essential. Otherwise, repeated errors by the same importer could mislead SISAM into believing that incorrect behaviour is correct. SISAM removes this bias on the fly, which is possible because of its fully Bayesian modelling approach.
Although it will not be easy, a careful plan is already in place to utilize deep learning and Large Language Models (LLMs) to process product descriptions within SISAM, without compromising the aforementioned advantages.
Moreover, the tool will have to be adjusted to take into consideration a major change in the way goods are described by importers. As explained in the 103 edition of WCO News, a catalogue describing products using pre-defined attributes has been introduced in SISCOMEX. Called the Product Catalogue (PC), it allows the importer to describe the characteristics of their goods in a standardized manner (using structured fields). This facilitates the reuse of information for future transactions, reduces the number of errors in filling out declarations, and speeds up the analysis of operations. The use of this catalogue is about to become mandatory. To fully benefit from the structured fields, we will need to modify SISAM machine learning models. These changes are under development.
Helping well-intentioned users
SISAM was designed to detect errors in classification codes, not to assist well-intentioned importers and exporters in classifying goods. For that purpose, a new system called CLASSIF[1] was created. This system analyzes the classification of goods using NCM codes, which are based on Harmonized Commodity Description and Coding System (HS) codes, with two additional digits. Importers and exporters can enter the description of a good in the system, which will then show them information such as the NCM codes which contain the words provided, and the legal notes related to these codes, as well as the WCO Explanatory Notes to the WCO Harmonized System, advance rulings issued for these codes, and classification suggestions that come directly from SISAM.
In fact, we have adapted SISAM to estimate probabilities based solely on the description of the goods, bypassing other attributes it normally uses. This makes the model somewhat weaker, but comes with the advantage of not instructing users on how to fool SISAM. If an ill-intentioned importer keeps changing a description until CLASSIF suggests the code he would like to use, this does not mean SISAM will not catch the error later. The result for importers remains satisfactory.
A recent article published in the World Customs Journal[2] presented a list of goods to be used to test the capabilities of Customs tariffs assistive systems to find correct HS codes, with each good presenting specific difficulties for the systems. We translated the list into Portuguese and entered them into CLASSIF. The results are shown in Table 1. It is important to mention that CLASSIF was programmed to suggest 8-digit codes when the quality of the result is considered high, and only 4-digit codes when it is low.
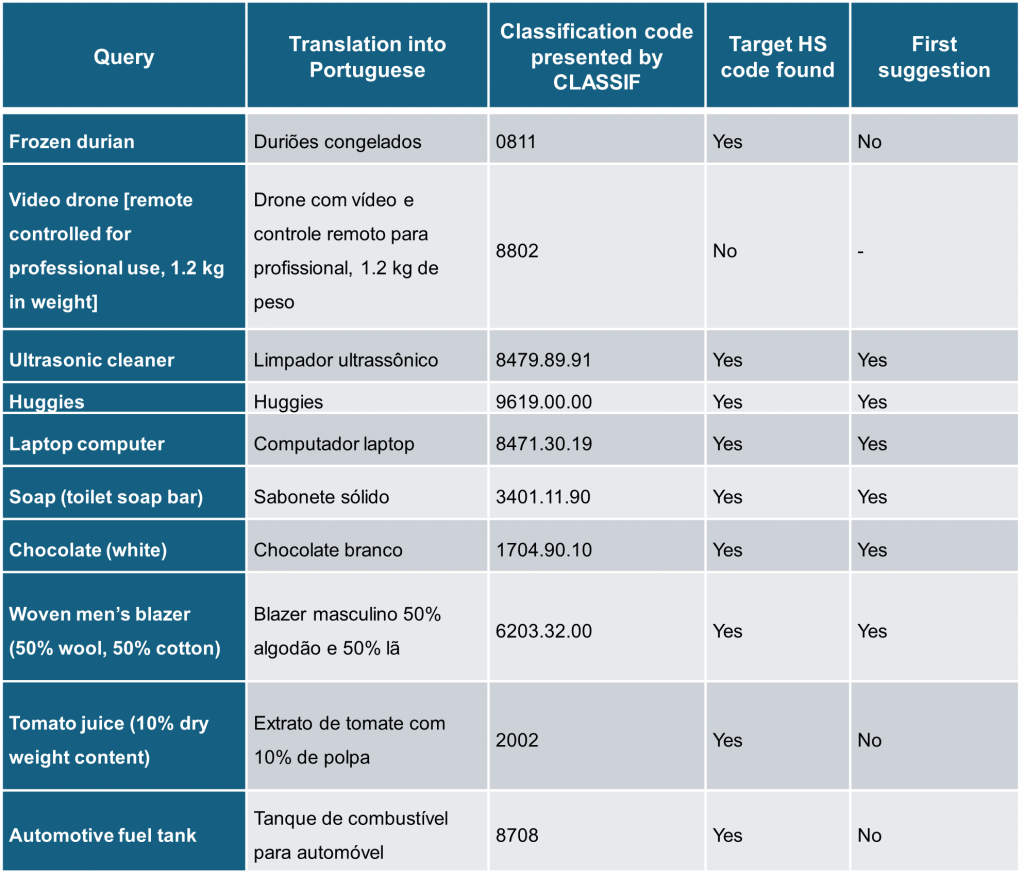
Every time CLASSIF proposed an 8-digit code, the result was correct, and the correct code was the code proposed by the system as having the higher probability of accuracy. That happened in six out of ten trials. On three occasions, CLASSIF proposed a correct 4-digit code not as the first most probable result, but never among more than ten alternatives. Once, CLASSIF failed completely. We have identified the cause as “garbage” inside SISAM’s knowledge base, which will soon be removed.
Supporting Post-Clearance Audit (PCA)
SISAM was initially developed to assist in the selection of import declarations for inspection at primary zones. However, its risk reports can be grouped in various ways, such as by company or tariff classification. Such aggregations of data can be useful to the teams in charge of PCAs and contribute to shifting Customs controls to the post-clearance phase, in line with trade facilitation agreements. Currently, instead of developing aggregation mechanisms within SISAM, we are choosing to feed our data lake with SISAM’s individual reports which can be aggregated using software like Power BI, CONTÁGIl[5], ANALYTICS[6], Jupyter Notebook, HUE and SPARK.
Using lessons learned to develop other new systems
Several new systems have been introduced to further strengthen the country’s ability to manage and monitor international trade and passenger movements.
A single window environment
Brazil is currently developing a new foreign trade single window system, known as the Portal Único do Comércio Exterior (PUCOMEX).[7] This system streamlines and centralizes the processing of trade-related documentation, offering substantial benefits for traders, particularly in terms of reducing the time and costs associated with cross-border operations. For Customs administration, PUCOMEX provides critical advantages in overseeing and managing international trade flows. The centralized platform allows for more efficient monitoring and improved coordination among various governmental agencies involved in Customs and border control.
The development of PUCOMEX has been shaped by the successful experiences of internally developed Customs software, such as ANIITA and PATROA. Although PUCOMEX is being developed by an external company, Serpro (Federal Data Processing Service), it draws extensively on the knowledge and expertise acquired from these internal projects. This approach has proven highly beneficial, enabling agile experimentation before transitioning to a more robust system, with a dedicated company engaged to develop and maintain the new platform.
Integrating Customs and airlines systems for passenger control
Our traveller control system is now integrated with airline systems and assesses risks considering the economic status of the travellers, the chosen itinerary, prior travel, and undeclared, but repeated, flight companions.[8],[9] Face recognition tools are also used across airports and directly connected to Customs systems.
Monitoring vehicles
A system called SIVANA monitors vehicles near Brazil’s land borders by leveraging images taken by road surveillance cameras. It allows Customs officers to analyze the historical routes of target vehicles and receive real-time information on their location, enabling them to respond promptly to potential threats, particularly narcotics trafficking and the trade in counterfeit products.
To enhance the capabilities of SIVANA, particularly in identifying patterns and anomalies that could indicate illicit activities at Brazil’s land borders, we partnered with Itaipu Parquetec, an innovation ecosystem that integrates entities such as educational institutions, companies and government agencies. The objective is to develop an application called SMART WALL, using Bayesian analysis and deep learning.
Ensuring consistency of data across all trade documentation
Another example of an innovative technology is the BATDOC system[10], created to compare import declarations (which are electronic documents meant to be understood by a computer) with supporting documents which are still provided as digital documents or scanned images of original forms, such as invoices and bills of lading. This system helps ensure data consistency across all documentation. Utilizing optical character recognition (OCR) technology, BATDOC automatically compares key information, such as the names and addresses of the parties involved, as well as the quantities and values of goods. By cross-referencing these documents, BATDOC helps to control the errors and discrepancies, thereby enhancing the accuracy and reliability of the information.
Leveraging external expertise to automate X-ray image analysis
One key limitation of SISAM was its inability to incorporate X-ray images into its analysis. To address this gap, the AJNA project was launched. AJNA[11] is a system powered by a deep neural network, which collects, displays, and conducts an automated analysis of X-ray images.
Initially, AJNA was operated exclusively at the Port of Santos, one of the largest ports in Latin America. The system managed processes such as gathering images from bonded warehouse scanners, storing these images in a centralized and standardized manner, and cropping the images to isolate containers from the trucks carrying them. Additionally, AJNA could detect cargo in containers that were supposed to be empty.
Like SISAM, AJNA was designed to be developed and maintained by an internal team. However, while SISAM was the sole artificial intelligence project being developed at the time, AJNA was launched together with nearly 40 other artificial intelligence-related projects. Soon it became necessary to leverage external expertise to develop the application further. Two projects are currently being carried out with private companies.
Using X-ray images to detect classification errors
The first one is X-Class, a project aimed at using X-ray images to detect classification errors. We chose to work with a startup, believing that only a meticulously crafted deep learning model can handle all the peculiarities of the project, and because we have millions of images and corresponding import declarations to train a model.
The task is complex due to the sheer existence of over 10,000 codes. While some of these codes correspond to goods that produce distinct and easily recognizable patterns in X-ray images, others correspond to goods that produce similar ones. Complicating matters further, different goods are often packed in the same containers, leading to overlapping patterns that are difficult to distinguish. The challenge is exacerbated by the limitation of being able to use only a single-angle X-ray beam, making it even more difficult to accurately separate and identify the various types of goods within a container.
Fortunately, SISAM will send the results of its classification analysis to X-Class, reducing the number of probable codes to a handful. X-Class may still not able to recognize an item in some cases, but it will at least rule out SISAM’s suggestions which are incompatible with the observed images.
Leveraging Google Gemini for analysis of images of small parcels and passenger luggage
The second project relates to small parcels and passenger luggage. To help with the analysis of scanned images, we decided to leverage the Google Gemini model. This tool is pre-trained and multimodal, i.e. able to work with and analyze more than just text. When passed an image, Gemini can describe or answer questions about the content, summarize the content, and extrapolate from the content.
This approach eliminates the need to use data to train the model. Instead, Customs officers must enter questions into the system in Portuguese. There are two types of questions. Officers can ask the machine to detect a pre-defined item such as drugs, arms or wildlife items, for example: “Are there any drugs in this luggage?” or “Is there anything that looks like the skeleton of a bird in this package?” The second type of question is more sophisticated and can be used to detect tax evasion, for example: “Is there anything in this package that does not correspond to the provided description which was …?”
So far, only questions of the first type have been tested. The results are promising. X-ray images of small packages are much clearer than the images of containers and frequently speak for themselves, reducing the necessity for integration with other analytical tools.
Conclusion
The use of artificial intelligence, which until not so long ago was a novelty, has become widespread in Brazil’s Customs. Initial resistance, which stemmed from uncertainty and scepticism, has been overcome and been replaced by an overwhelming demand for solutions in the field of computer science. Our initial development model, which was based around only an internal team, had to be extended to include partners from universities, innovation parks, big techs, startups and the Federal Data Processing Service of Brazil. We are still learning to handle the new reality.
Artificial intelligence does not float around in space. It needs to be connected to a vast technological ecosystem that includes different data collection tools, multiple databases, data visualization tools, real-time alert systems, legacy systems, new internal systems, government systems outside Brazil’s Federal Revenue Secretariat and private companies’ systems. Such integrations frequently represent a significant part of development efforts.
Our artificial intelligence systems learn from people, without trying to replace them, and all decisions made by these systems are reviewed. Whenever possible, our systems produce explanations to help such reviews.
Artificial intelligence has become indispensable in managing the complexities of modern trade. While significant results have already been achieved, including more accurate and efficient processing, there remains vast potential for future advancements.
More information
jorge.jambeiro@rfb.gov.br
gustavo.coutinho@rfb.gov.br
kelly.morgero@rfb.gov.br
[1] Jambeiro Filho, Jorge. Artificial Intelligence in the Customs Selection System through Machine Learning (SISAM). Prêmio de Criatividade e Inovação da RFB, 2015. https://www.jambeiro.com.br/jorgefilho/sisam_mono_eng.pdf
[2] Coutinho, Gustavo. Aniita – uma abordagem pragmática para o gerenciamento de risco aduaneiro baseada em software. Prêmio de Criatividade e Inovação da RFB, 2012. http://repositorio.enap.gov.br/handle/1/4607
[3] Domingues, Luiz Henrique; Navarro, Claudia Elena Figueira Cardoso; Steckel, Carlos Humberto; Lima, Lucas Araújo de; Casado, Marco Antônio Rodrigues. Prêmio de Criatividade e Inovação da RFB, 2023. https://ea.ufba.br/wp-content/uploads/2023/11/E-book-A_22-premio_16_abr24.pdf
[4] Grainger, Andrew. Customs Tariff Classification and the Use of Assistive Technologies. World Customs Journal, 2024.
[5] Figueredo, Gustavo Henrique Britto. Um novo paradigma na auditoria em meio digital. Prêmio de Criatividade e Inovação da RFB, 2010. https://repositorio.enap.gov.br/handle/1/4580
[6] Receita Federal do Brasil. Receita Federal apresenta ferramentas de gerenciamento de riscos em evento informal da OCDE na Suécia. 2024.
[7] Corbari, Jackson Aluir; Zambrano, Alexandre da Rocha; Morgero, Kelly Cristina Silva. Portal Único Siscomex: A transformação digital no comércio exterior brasileiro. 2024.
https://alfandegas.cplp.org/Newsletter/Documents/Newsletter%208%20janeiro%202024_VF.pdf
[8] Moraes, Felipe Mendes. Sistema e-DBV – Módulo viajante único, 2017. https://repositorio.enap.gov.br/handle/1/4635
[9] Thompson, Ronald Cesar. Projeto IRIS – Reconhecimento facial de viajantes. 2016. https://repositorio.enap.gov.br/handle/1/4627
[10] Barbosa, Diego de Borba. Batimento Automatizado de Documentos na Importação – BatDoc. Prêmio de Criatividade e Inovação da RFB, 2016. http://repositorio.enap.gov.br/handle/1/4630
[11] Brasílico, Ivan. AJNA – Plataforma de Visão Computacional e Aprendizado de Máquina. Prêmio de Criatividade e Inovação da RFB, 2017. https://repositorio.enap.gov.br/jspui/handle/1/4634